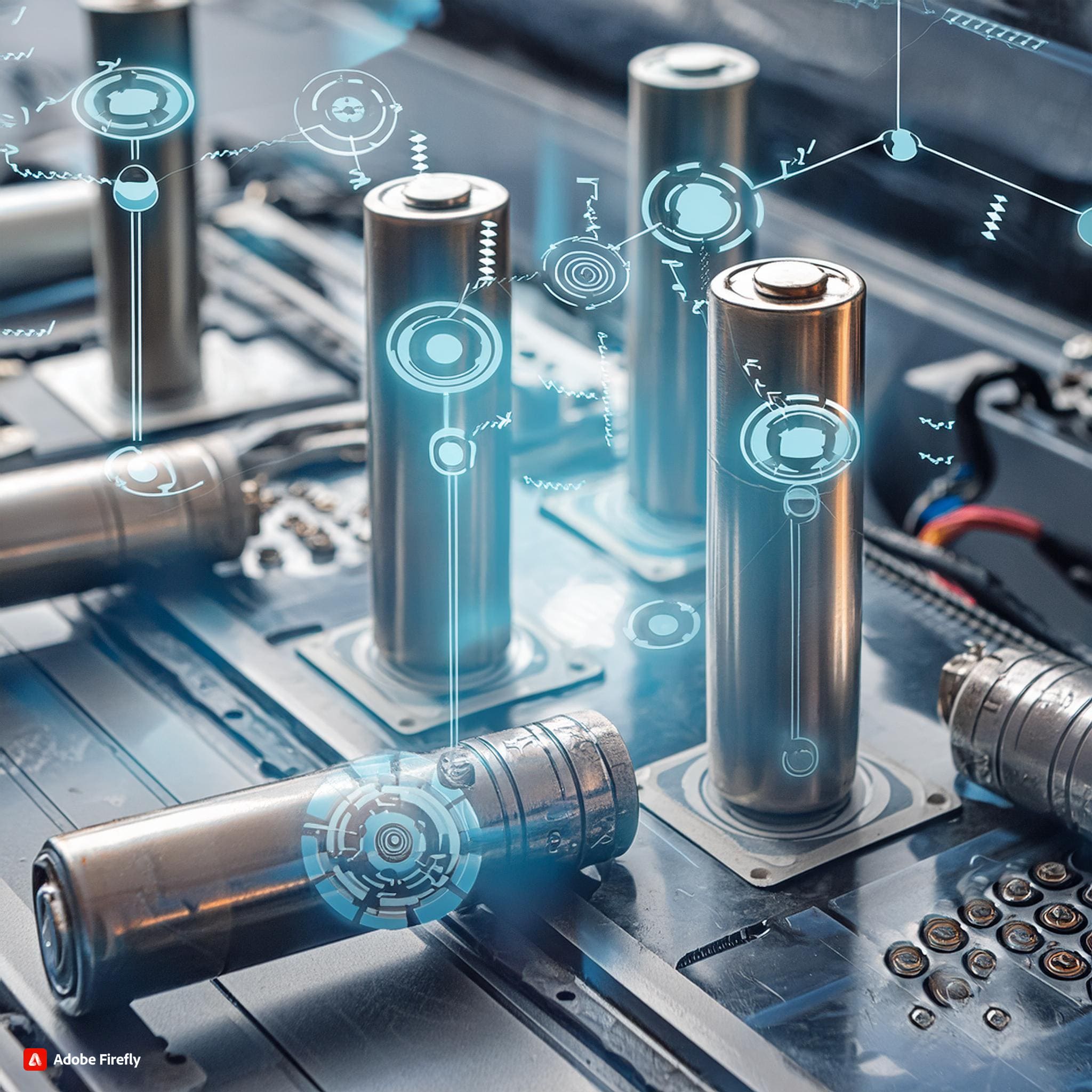
SENSIGA
Intelligent operando measurement for advanced BMS and AI for aging prediction
Overview
Battery cell monitoring using optical fiber sensors, multi-physics instrumentation, and artificial intelligence for degradation estimation and aging prediction.
Dr. Vincent HEIRIES (CEA LETI / CEA LITEN)
Prof. Jean-Marie TARASCON (Collège de France)
The recent convergence of battery science and optical sensor engineering opens up new opportunities for battery diagnostics. We aim to develop ultra-sensitive optical sensors to monitor the physical-thermal and chemical parameters of batteries under real-world conditions. We are also exploring battery monitoring (Li-Ion, Na-Ion, Solid State) using multi-physics sensor systems (thermal, acoustic, mechanical, electrical) to extract observable degradation signatures and enhance the predictive capabilities of battery management systems (BMS). This monitoring could increase the reliability, lifespan, and reduce the cost of batteries. Our research program combines the design of specific sensors with signal processing approaches based on artificial intelligence to diagnose and optimize battery performance.
Keywords
Sensors, optical sensors, BMS, state estimators, algorithms, artificial intelligence
Related news
Pas d’actualités
Tasks
Our research
Functional optical sensors integrated into the core of battery cells
The first objective is the in-depth exploration of internal cell instrumentation methods using optical fiber sensors. We will generalize coupled temperature and pressure detection to monitor SEI formation during cell formation, thereby establishing a reliable methodology for manufacturers. We will refine optical calorimetry to determine the specific heat capacity of cells, which is crucial for the design of cooling systems. The birefringence of Bragg gratings will be used to monitor mechanical stresses and cracks in electrodes, with this approach extended to all-solid-state batteries. Optical multiplexing will enable the creation of thermal and stress imaging to identify potential incidents. Chemical compound detection will use sensors exploiting evanescent waves and operando infrared measurements via chalcogenide optical fibers. Finally, multiplexing, which transmits multiple pieces of information simultaneously via a single optical fiber, will significantly enhance the estimation of charge state, health state, and power state of cells.
Artificial intelligence and physics-informed deep learning for exploiting sensor data integrated into battery cells
The second objective focuses on processing data from intra-cell and extra-cell sensors, and the development of signal processing algorithms for battery state diagnostics and aging prognosis. By leveraging the “over-instrumentation” of cells, this project aims to enhance the understanding of internal physicochemical mechanisms and improve the performance, longevity, and safety of batteries. Experimental data from internal and external sensors will help identify the most effective sensors and processing algorithms for each objective and quantify their performance. The artificial intelligence algorithms developed will significantly enhance BMS functionalities and promote optimal battery management.
The consortium
4 academic laboratories, 2 CEA institutes
Multi-sensor monitoring combined with advanced artificial intelligence methods will revolutionize our understanding of batteries. The SENSIGA project will develop multi-physics sensor systems, both intra-cell and extra-cell, and establish a multi-physics database on battery aging. These innovations will not only deepen our knowledge of parasitic reactions, degradation phenomena, and their interactions but also create advanced AI methods to predict their evolution. This will equip next-generation battery management systems (BMS) with exceptionally enhanced predictive capabilities, thereby optimizing battery performance and longevity.
Thanks to the sensor systems developed within the project and the effective diagnostic and aging prognosis methods based on AI, we will truly unlock battery eco-design, enhance the most impactful stages of manufacturing, and avoid over-design. These advancements will also optimize the BMS of current and future generation batteries, reducing their aging and, consequently, their carbon footprint throughout their lifecycle. The developed methods could also enable optimal end-of-life management, facilitating the most appropriate repurposing or recycling of batteries.
Training of 5 doctoral students and 8 postdoctoral researchers.
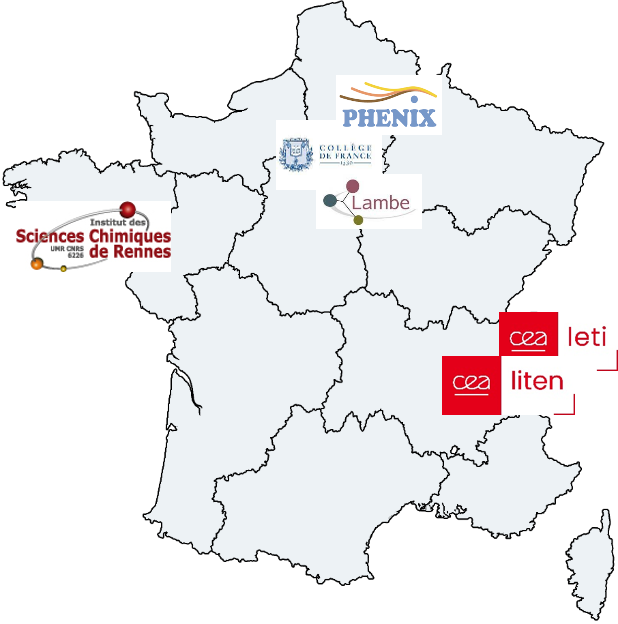
Les autres projets PEPR
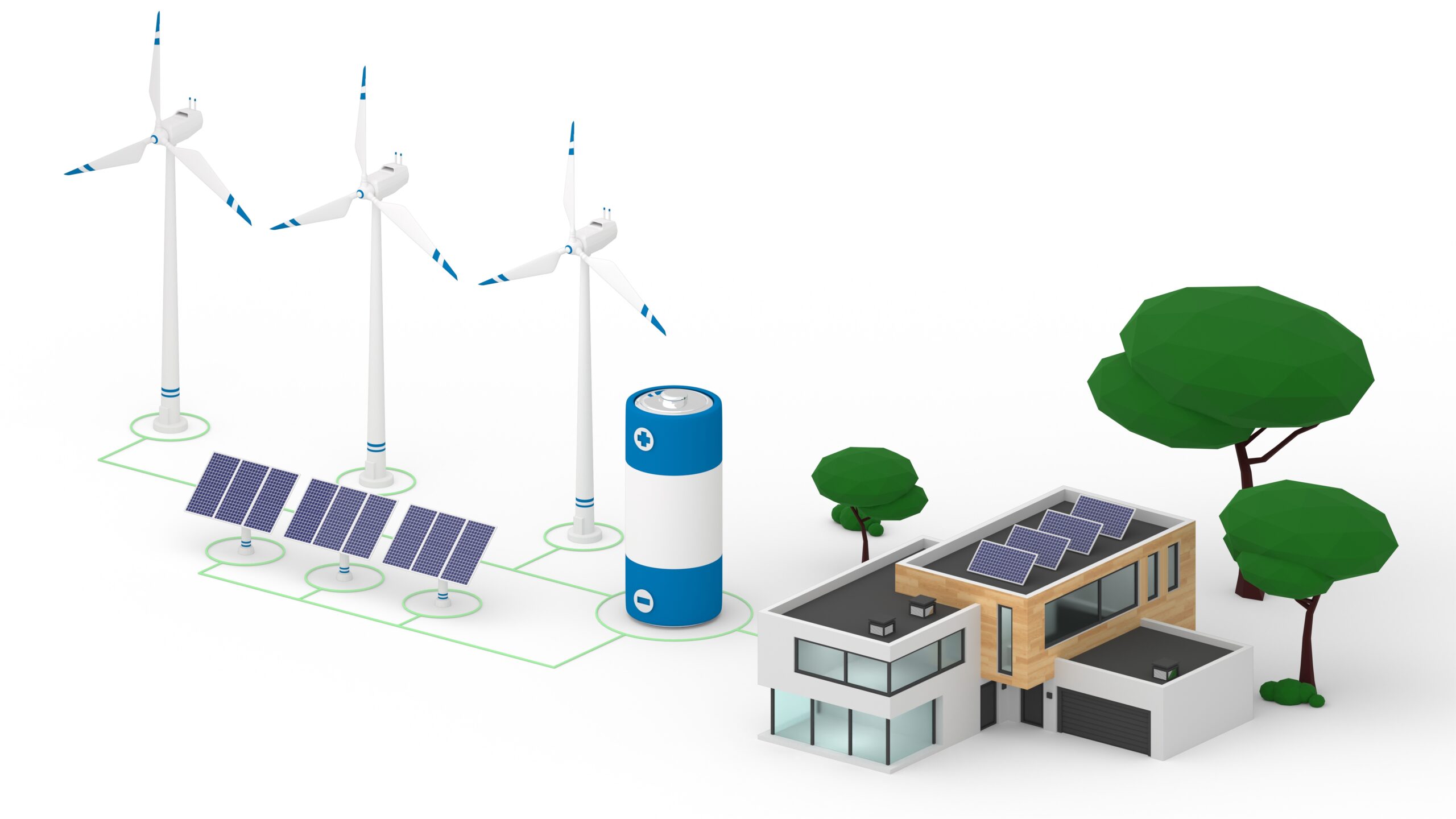
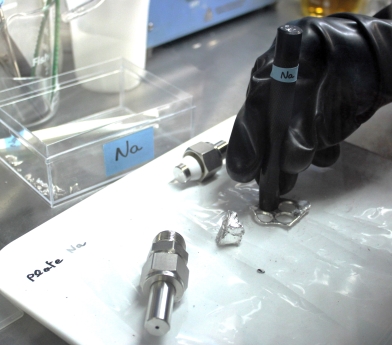
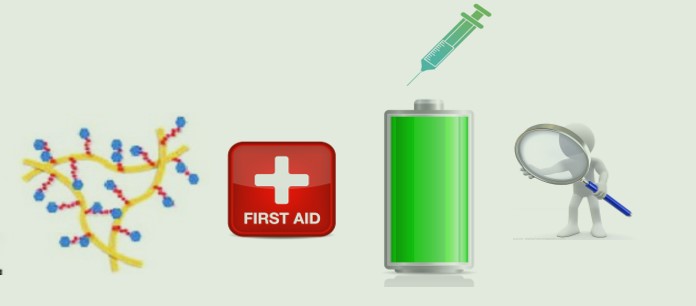
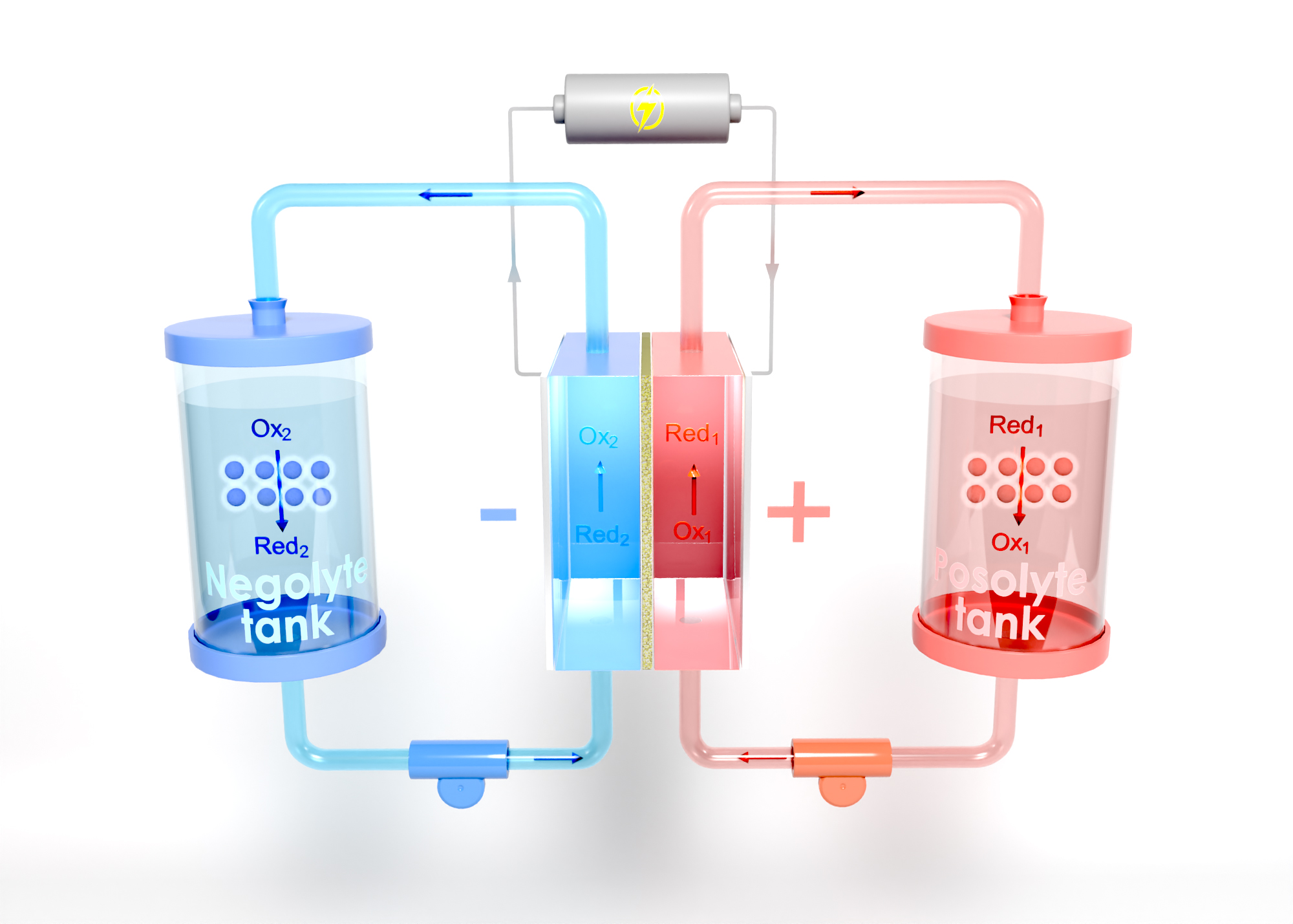
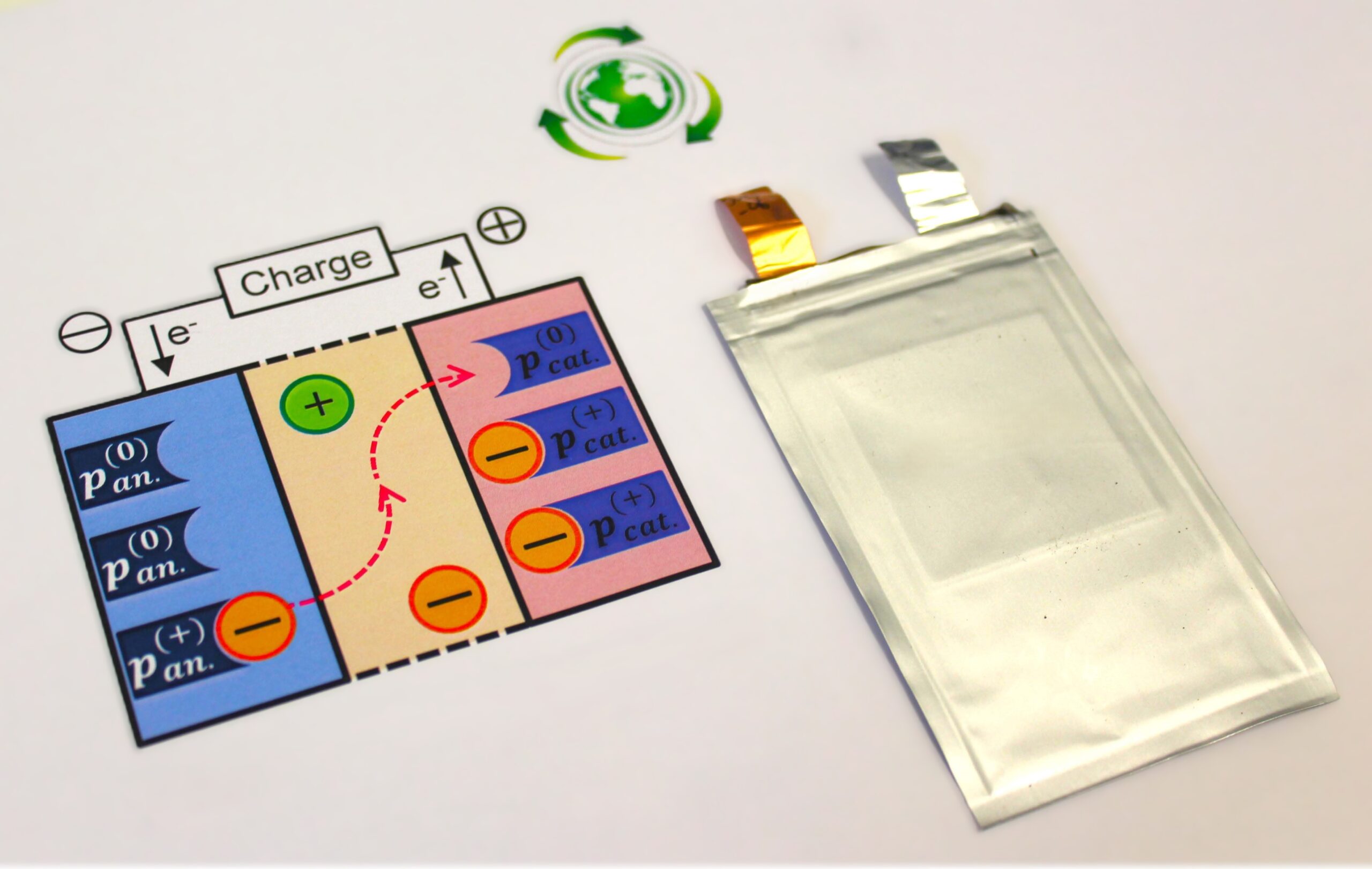
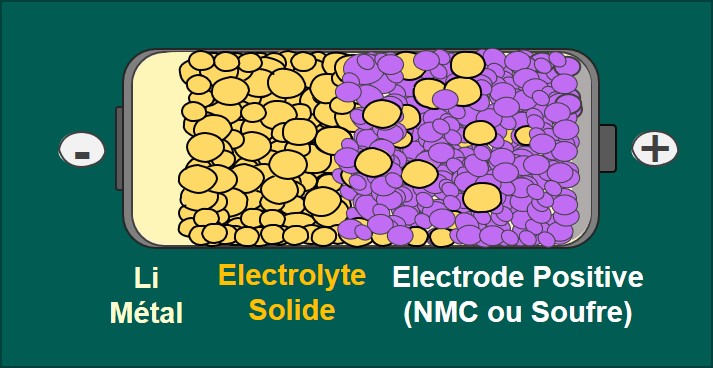