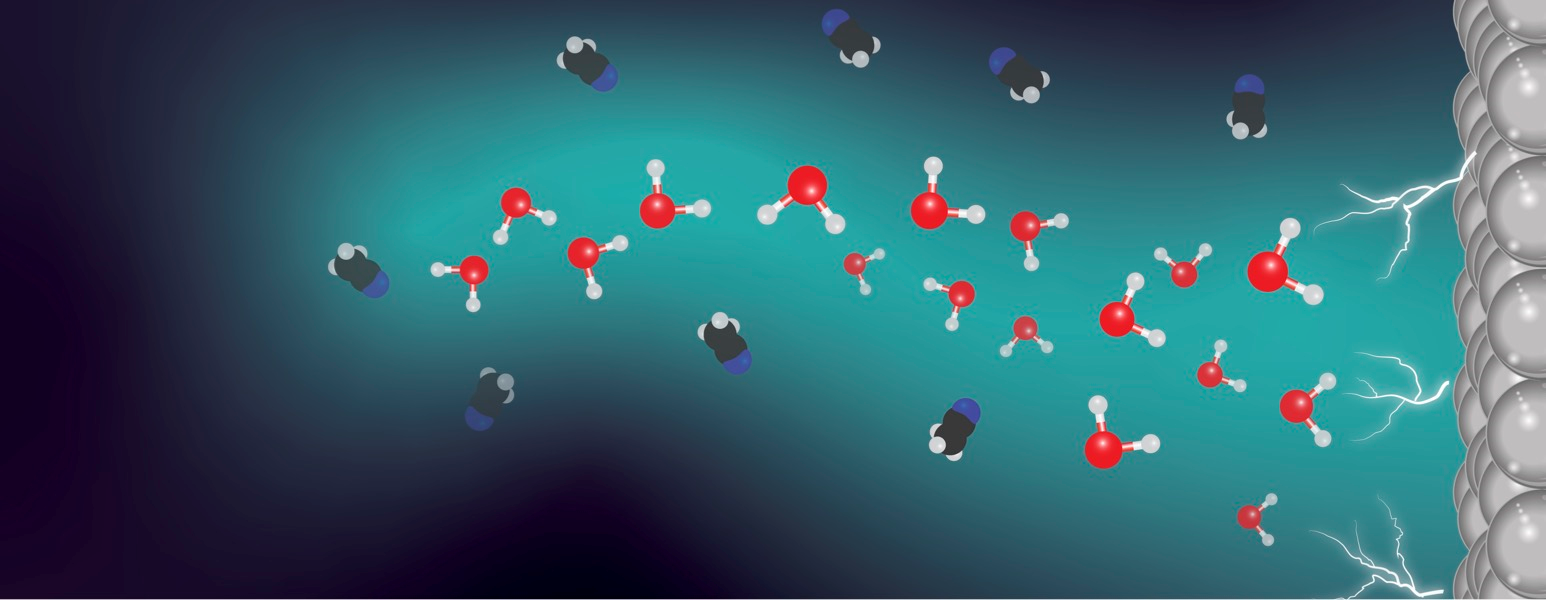
BATMAN
Combine artificial intelligence with physical models to develop new batteries
Overview
Data exploration, artificial intelligence, and digital twins for the next generation of batteries
Prof. Mathieu Salanne (PHENIX, Sorbonne Université / CNRS)
Prof. Alejandro Franco (LRCS, Université de Picardie Jules Verne / CNRS)
Research on electricity storage increasingly relies on numerical simulations, typically based on physical principles. BATMAN aims to develop new models that integrate data science and artificial intelligence approaches. Three main objectives are targeted: finding optimized electrolytes and materials, understanding chemical reactions at battery interfaces, and optimizing manufacturing processes. The project also aims to create a digital twin to predict battery performance based on the properties of the manufactured electrodes.
Keywords
Multi-scale simulations, artificial intelligence, digital twins, high-throughput screening, high-performance computing, electrolytes, materials, manufacturing processes
Actus en lien
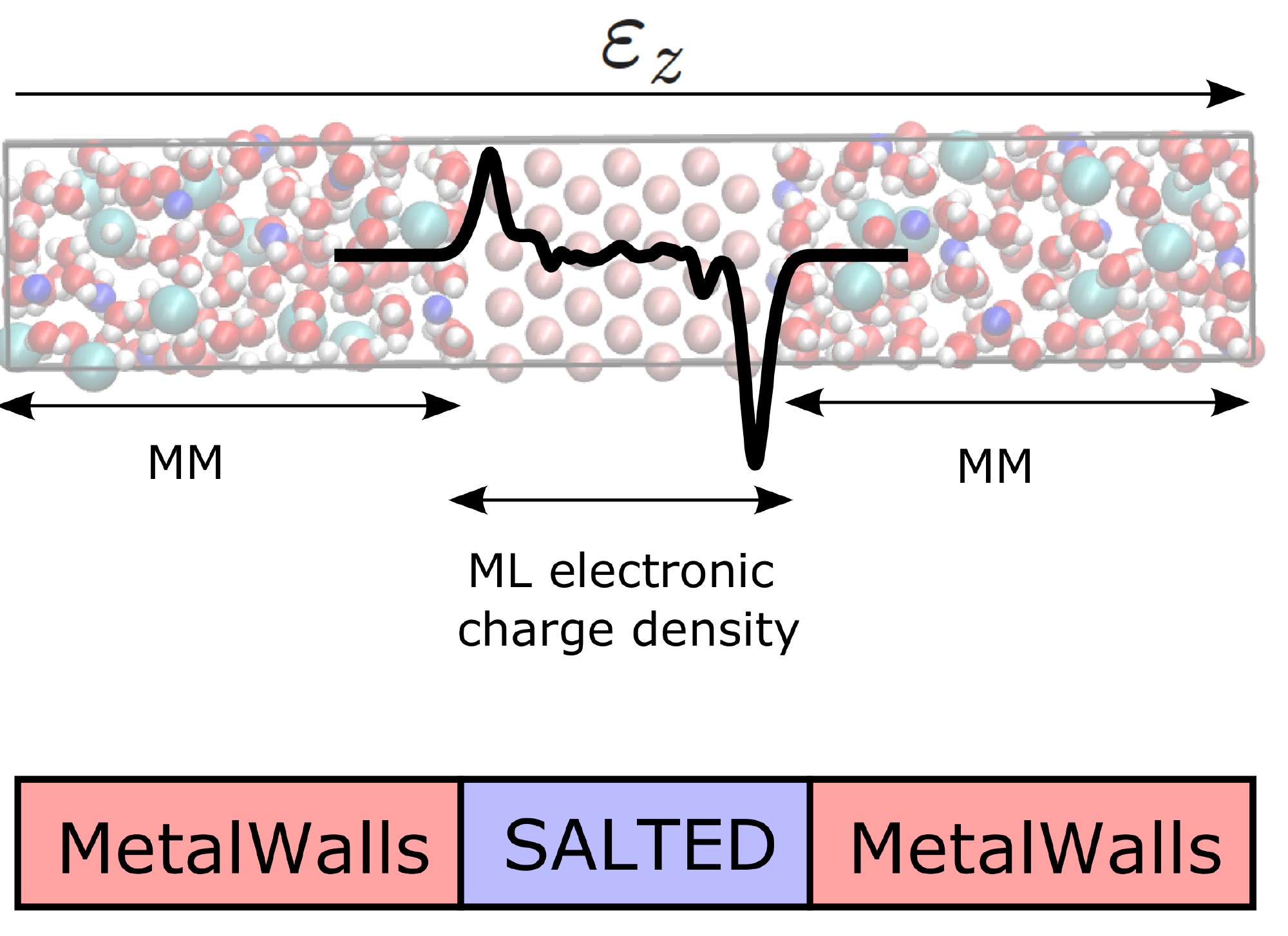
Pas d’actualités
Tasks
Our research
Screening of new materials for next-generation batteries
The first objective is “high-throughput screening,” which aims to identify optimized electrolytes for next-generation batteries and materials suitable for high-power devices. Well-established techniques, such as electronic structure calculations and molecular dynamics simulations, will be used to explore large material databases, whether these materials have been synthesized or not. This will provide lists of material compositions with improved performance for batteries.
Studying battery interfaces with machine learning approaches
The second objective focuses on understanding the chemical reactions occurring at battery interfaces. New machine learning-based tools will be developed to simulate changes at the electrode/electrolyte interface, incorporating electronic structure effects into molecular dynamics simulations. These methodological advancements will enhance the understanding of phenomena such as dendritic growth in all-solid-state batteries and electrolyte decomposition on Na-ion battery electrodes.
Digital twins for battery manufacturing processes
The third objective is to develop a “digital twin” to optimize battery manufacturing processes. Battery electrodes are composed of blends of different materials, each with a specific role. To improve performance, it is crucial to optimize the textural properties of the composite and the interfaces between materials. A digital twin will be created to predict battery performance based on the properties of the manufactured electrodes.
The consortium
5 academic laboratories, 2 CEA institutes, IFPEN, and CINES
The use of numerical simulations and artificial intelligence opens up exciting new perspectives for energy storage research and the development of higher-performance batteries. Beyond the results obtained concerning electrode materials and electrolytes, BATMAN will enable the development of numerous digital tools for high-throughput screening, advanced interaction potentials, and digital twins that can be reused in other contexts, particularly on French supercomputers.
Numerical simulations drastically reduce the number of experiments needed to develop new materials. The optimization of manufacturing processes through digital twins enables significant savings in energy consumption and the use of critical materials.
Training of 5 doctoral students and 7 postdoctoral researchers
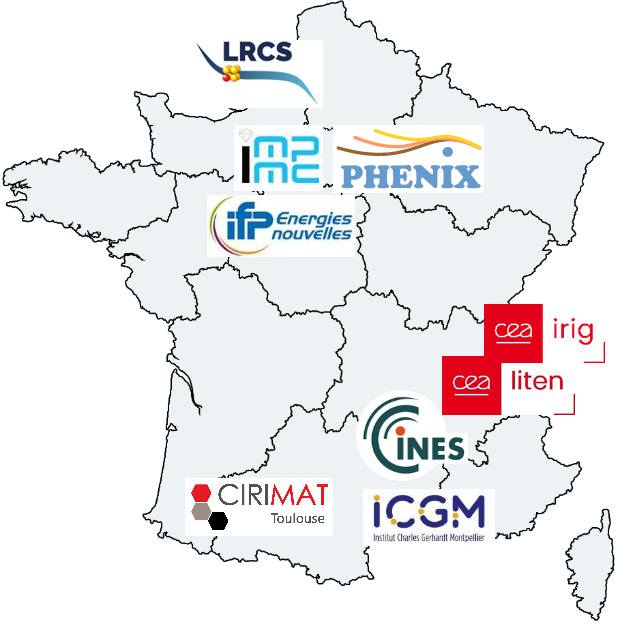