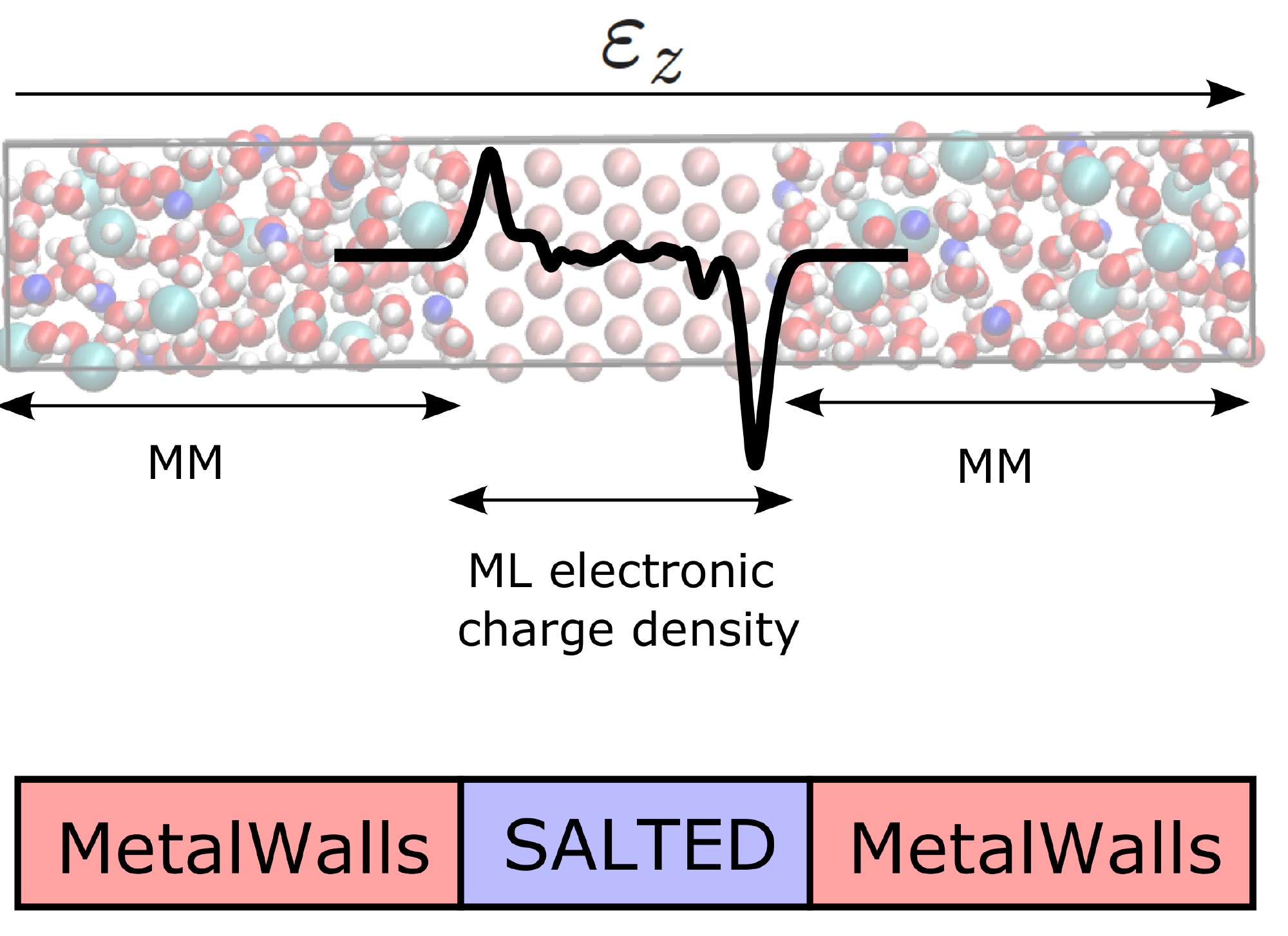
New machine learning model for electrochemical interfaces

August 26, 2024

The BATMAN project publishes its first results on the simulation of electrochemical interfaces.
Andrea Grisafi and Mathieu Salanne
The computational study of energy storage materials requires simulating the interface between a metallic electrode and a liquid electrolyte solution on nanoscale time and length scales. While interactions between electrolyte atoms can be easily calculated using classical models, accurately reproducing the electronic charge distribution at the electrode surface requires quantum mechanical treatment. However, due to the extremely high computational cost needed to solve the quantum mechanical problem at each step of the simulation, integrating a consistent description of the electrode’s charge remains a major challenge for the currently available computational methods. In this context, one of the main objectives of the BATMAN project is to overcome this methodological challenge.
Indeed, a promising strategy to circumvent the computational cost of quantum mechanical calculations involves adopting machine learning techniques capable of predicting the electronic charge density at the electrode surface. From a technical standpoint, the greatest challenge lies in addressing the complex three-dimensional nature of the electronic density and its response to electrostatic perturbations caused by electrolyte atoms located arbitrarily far from the electrode surface. Recently, a machine learning method dedicated to predicting electronic density, known as SALTED, has been successfully used to achieve this goal by incorporating both the spatial symmetries of the quantum charge distribution and its non-local characteristics into the model [1].
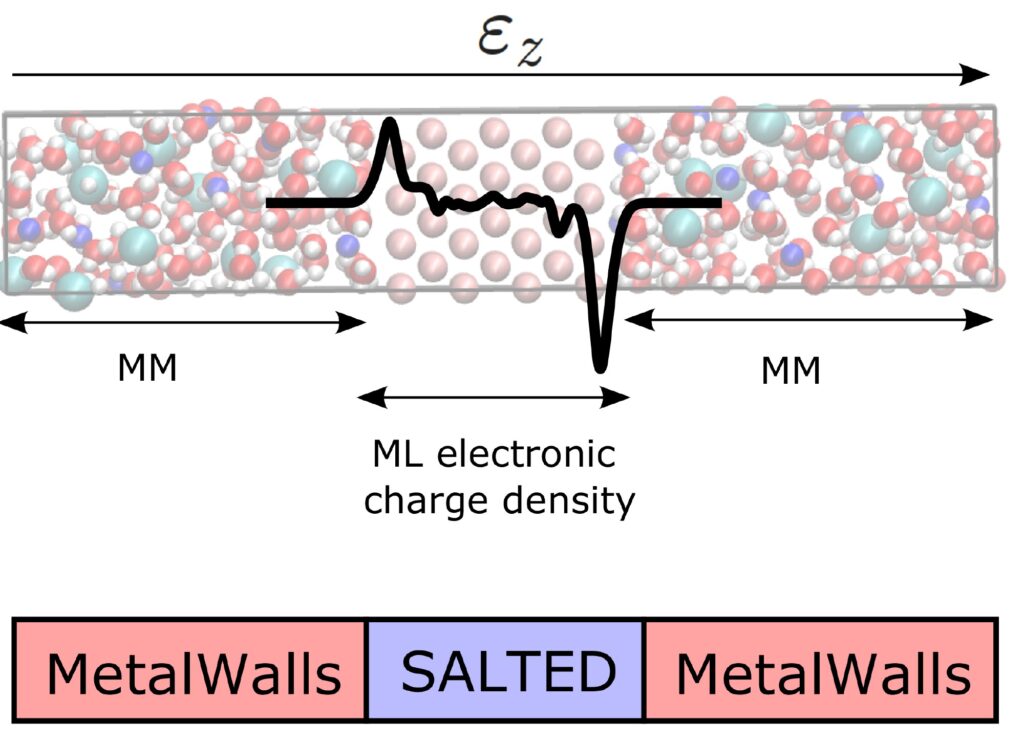
The SALTED method has been integrated into a classical simulation software for electrochemical systems developed at the PHENIX laboratory, MetalWalls [2]. At the core of this study is the calculation of the quantum electric field acting on the electrolyte atoms, as generated by the charge density of the electrode predicted by machine learning. The method was tested on a model of an ionic capacitor consisting of a gold electrode in contact with a concentrated aqueous sodium chloride solution (Figure 1). Thanks to the developed software interface, it was possible to achieve the unprecedented goal of simulating the atomic distribution of the electrolyte at the metal surface over several nanoseconds while maintaining the level of accuracy in the electrode’s charge required by quantum mechanics (Figure 2).
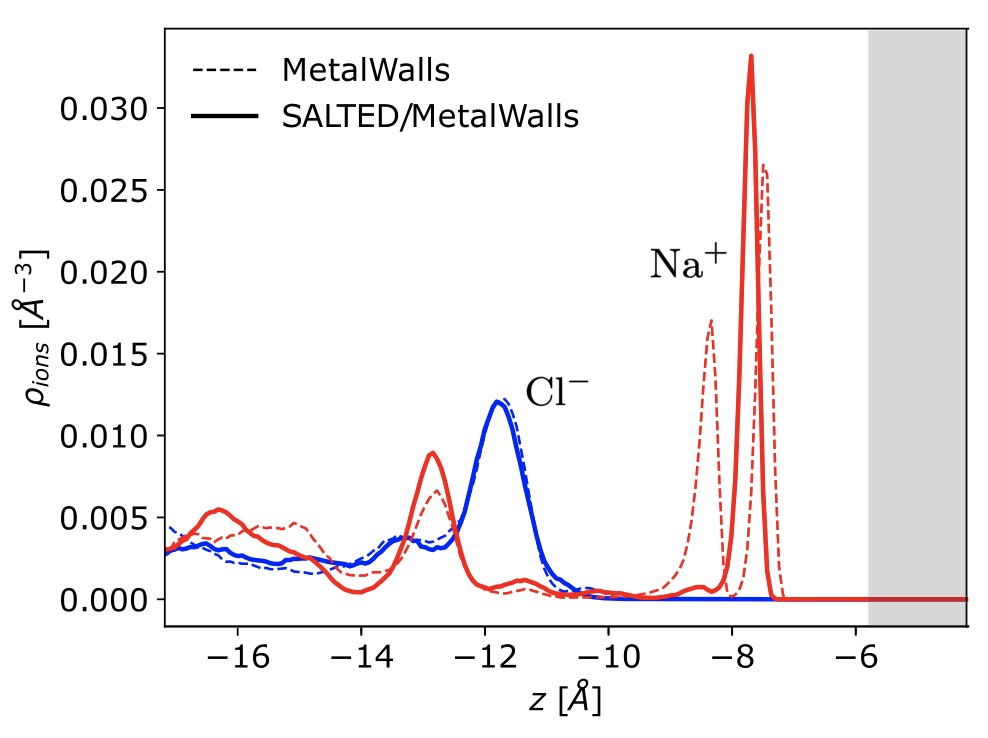